How We Taught Cloud To Deliver Products To The Shelves
Goods Checker is the name of our IT solution that serves for recognition of goods on store shelves. I would like to tell how the idea came about, what obstacles we had to overcome, and how it can help merchandizers.
IDEA: MERCHANDISING CAN AND SHOULD BE AUTOMATED
Every day in any modern store, long before the opening, work is in full swing. Many people are engaged in the layout of goods, using special schemes for goods layout and photos of shelves for reporting. Correct product placement on shelves is a significant part of manufacturers’ marketing strategies.
We noticed that there is a lot of manual labor in the process of controlling the product layout, and saw this as an opportunity for automation. Then we figured out how to transfer the entire routine work to the machine, leaving the decision-making to humans, so that the business process would become quick and easy.
WHAT TECHNOLOGIES ARE AT THE CORE OF THE PRODUCT?
Goods Checker is an artificial intelligence-based cloud service that analyzes the placement of goods on store shelves. The solution uses Machine Learning models, including neural networks and big data, which yields fantastic results in terms of speed and accuracy:
We use cloud technologies to process photos. After the application captures a photo, it analyzes the image using a specified algorithm. As it takes only a few seconds to analyze one image, we can process a large number of photos in a short time. For example, for one of the categories, we achieved a detection accuracy of 98%, a classification accuracy of 93%, and the recognition speed was from 5 to 15 seconds.
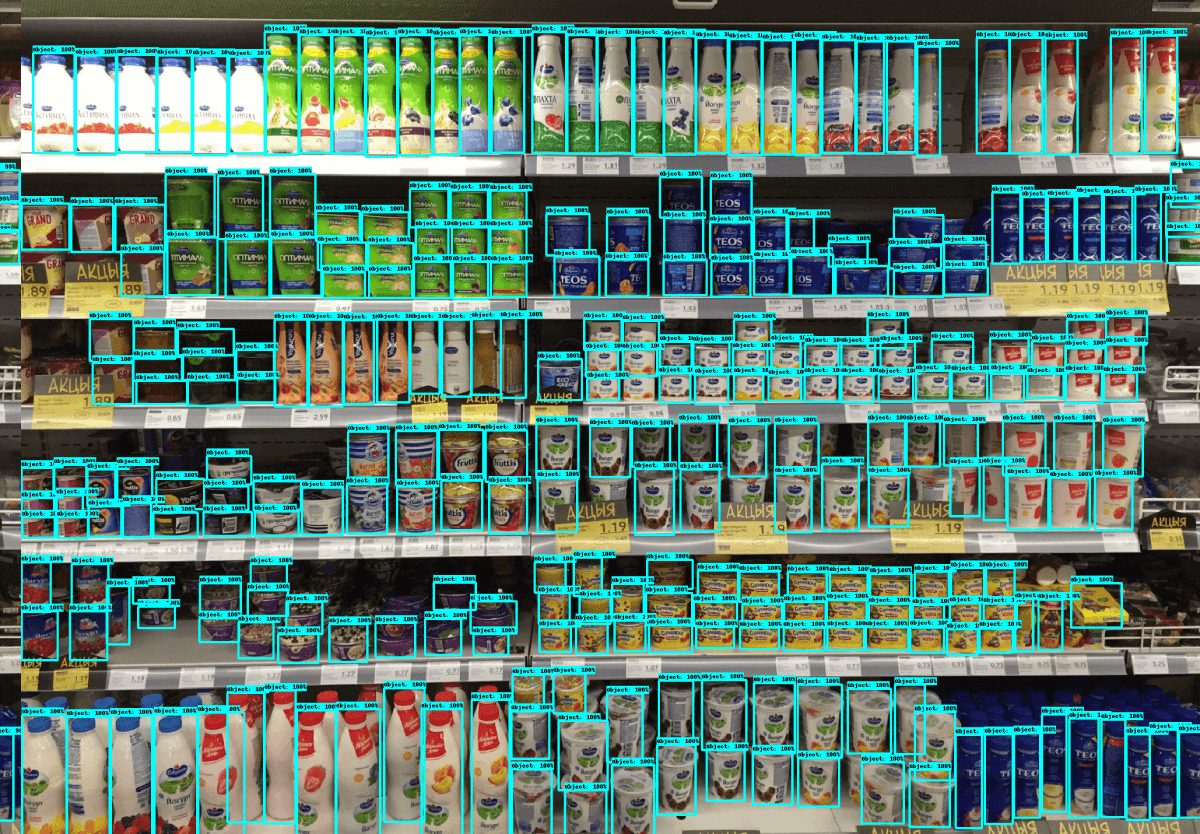
HOW DOES GOODS CHECKER HELP MANUFACTURERS AND MERCHANDISERS?
Thanks to Goods Checker, a merchandiser can immediately see what he or she did wrong. The application shows, which products are in their right places and which should be rearranged.
Goods Checker automates the process of working with the display of goods. The process includes numerous repetitive operations by several people, including merchandisers, sales representatives, and marketers.
Our service minimizes the human factor, reduces the processing time for photographs, and increases the accuracy of the information provided by field workers. The solution increases the number of sales and reduces the time for an audit of outlets due to relevant and timely statistics.
As a result, the merchandisers are sure that they did their job efficiently, and the photo bears a mark that serves as a proof of the correct placement. The application minimizes misunderstandings between employees and helps merchandisers maintain high quality and earn bonuses.
Specialists from the manufacturer’s side receive detailed analytics on the actions of the merchandiser, on how the goods are presented on the shelves, and what are the results of the product distribution in stores. The solution can recognize goods throughout the country, in any city and store, where a company sells relative products.
WHAT DIFFICULTIES DID YOU FACE?
The most time-consuming part of creating the service was preparations for training a neural network. For example, at the first stages, we had to mark by hand about 90 thousand products within 45 days. This was necessary to achieve the required accuracy of recognition for each commodity item.
Another challenge was the quality of photographs taken by merchandisers. Quite often, merchandisers get such poor-quality pictures of the shelves that it is difficult to even for a human eye to distinguish products on them, not to mention recognition by a machine. Sometimes, it is not possible to take a high-quality photo. In some cases, the lighting is poor, the passage is so narrow that one cannot move away to take a general picture. Therefore, we trained the system to analyze images with many defects. The solution can create an image from several fragmented photos of shelves, aligning the layout.
A quick change of the assortment of goods in stores was also a challenge. While the neural network was learning to recognize certain products, the manufacturer could put completely different ones on store shelves. Therefore, we trained the neural network to adapt to the constant change of assortment.
WHICH CATEGORIES OF GOODS ARE BEST FOR ANALYSIS?
It turned out that goods in hard packaging are easier for the machine to recognize. These are bottles, boxes, cans, and the like. Soft goods are more difficult to recognize because they usually look different in photographs. For example, chips can be placed on a shelf or they can lie with other goods.
Goods Checker can be trained to recognize any category of goods. The application is already able to recognize alcoholic and non-alcoholic drinks, tobacco and dairy products, including milk, kefir, curds, and yogurts. In the near future, we plan to start recognizing goods from the checkout area.
WHAT OTHER PROCESSES CAN WE AUTOMATE IN RETAIL SECTOR?
Digital technologies are changing the retail sector before our very eyes. Self-checkout stores now use smart carts. This is something like regular shopping trolleys, only equipped with sensors and scanners. The smart cart helps generate a receipt during the purchase process. The customer has to pay for the goods at the checkout.
In stores, cameras are integrated with servers to collect additional customer information and do analysis. The smart camera signals when queues appear at the checkouts and it is time to allocate additional cashiers. It also collects data on clients’ age and gender, and the time when they visit the store. Analytical systems using conventional cameras are able to monitor voids on the shelves.
Our latest improvements to the solution include creation, copying, and downloading of planograms. A planogram is the rules for how products should be placed on store shelves.
We do not have to explain the benefits of automation any longer. In some large networks, the digitalization of business processes and of the sales areas is actively underway. Many stores have introduced self-checkout counters and RFID tags. Therefore, the retail industry is ready to take the next step and implement advanced IT solutions.